Predictive Maintenance in the Chemical, Oil and Gas Industry
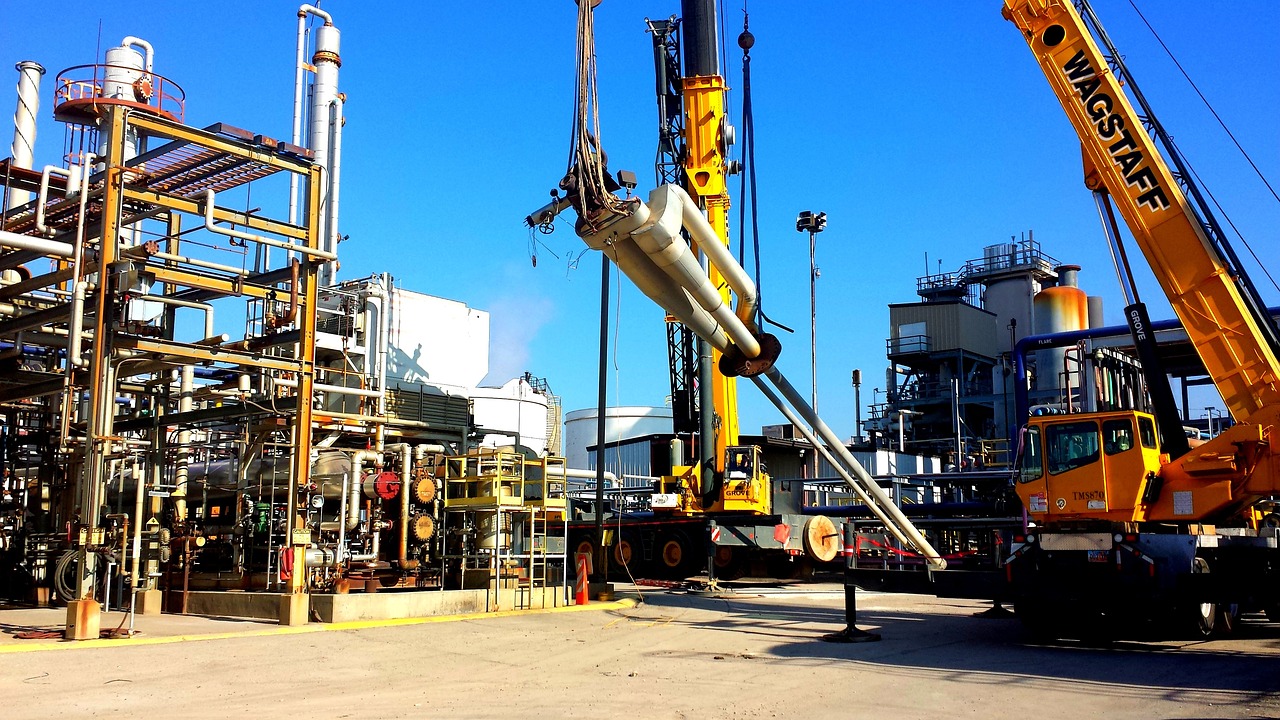
Predictive Maintenance in the Chemical, Oil and Gas Industry
Introduction
The chemical, oil, and gas industry is a cornerstone of the global economy, driving advancements in energy production, raw materials processing, and industrial applications. However, the sector is fraught with challenges, including equipment failures, unexpected downtimes, and safety risks. Predictive maintenance (PdM) has emerged as a transformative approach to addressing these issues, utilizing advanced technologies such as the Internet of Things (IoT), Artificial Intelligence (AI), and big data analytics to anticipate equipment failures before they occur. This blog explores how predictive maintenance is revolutionizing the industry by enhancing operational efficiency, reducing costs, and improving safety.
Understanding Predictive Maintenance
Predictive maintenance involves the use of real-time data and analytics to monitor the health of industrial equipment and predict potential failures. Unlike reactive maintenance (fixing equipment after failure) or preventive maintenance (routine maintenance regardless of equipment condition), PdM ensures that maintenance is performed only when needed, reducing unnecessary downtime and expenses.
Key components of predictive maintenance include:
- Sensors and IoT Devices: These collect real-time data on temperature, pressure, vibration, and other critical parameters.
- Big Data Analytics: Analyzing large volumes of operational data to identify patterns and predict failures.
- Machine Learning and AI: Algorithms that refine predictive models, improving accuracy over time.
- Cloud Computing: Storing and processing vast amounts of data for remote monitoring and analysis.
The Role of Predictive Maintenance in the Chemical, Oil, and Gas Industry
1. Reducing Unplanned Downtime
Unplanned downtime is one of the most significant challenges in the industry, leading to financial losses and production delays. By implementing PdM, companies can detect early signs of equipment deterioration and schedule maintenance at the most opportune times, ensuring continuous operations.
2. Enhancing Equipment Lifespan
Predictive maintenance extends the lifespan of critical assets by ensuring timely intervention before minor issues escalate into major failures. This proactive approach minimizes wear and tear, ultimately increasing the return on investment for industrial equipment.
3. Improving Safety Standards
Faulty equipment poses severe safety risks, including explosions, leaks, and environmental hazards. By leveraging predictive analytics, companies can detect anomalies that indicate potential hazards, allowing for timely corrective actions and ensuring compliance with safety regulations.
4. Optimizing Operational Efficiency
With real-time monitoring, companies can optimize energy usage, streamline maintenance schedules, and improve overall operational efficiency. PdM enables better resource allocation, reducing the need for excessive spare parts inventory and unnecessary labor costs.
Technologies Driving Predictive Maintenance
1. Industrial IoT (IIoT)
IIoT connects physical assets to digital systems, enabling seamless data collection and analysis. Smart sensors embedded in machinery continuously transmit performance data, allowing for predictive insights.
2. Artificial Intelligence and Machine Learning
AI-powered predictive maintenance systems analyze historical and real-time data to detect anomalies and predict failures. Machine learning models become more accurate over time, refining maintenance strategies for maximum efficiency.
3. Digital Twins
A digital twin is a virtual replica of a physical asset that simulates its performance under various conditions. Companies use digital twins to test predictive maintenance strategies and optimize asset management.
4. Edge Computing
Edge computing processes data closer to the source, reducing latency and enabling faster decision-making. In remote oil and gas facilities, edge computing ensures real-time analysis even in low-connectivity environments.
Case Studies of Predictive Maintenance in Action
Case Study 1: Offshore Oil Platform
An offshore drilling company implemented a PdM system with IoT sensors and AI analytics. The result was a 30% reduction in unplanned downtime and a 20% decrease in maintenance costs over two years.
Case Study 2: Chemical Manufacturing Plant
A chemical plant adopted predictive maintenance to monitor pump failures. By analyzing vibration patterns, they reduced pump failures by 40% and improved overall plant efficiency.
Case Study 3: Refinery Operations
A major refinery utilized digital twins and machine learning to optimize maintenance schedules. This reduced maintenance-related shutdowns by 25% and increased asset reliability.
Implementing Predictive Maintenance: Best Practices
- Invest in the Right Technology: Choose PdM solutions that integrate seamlessly with existing infrastructure.
- Start Small and Scale Gradually: Begin with pilot projects and expand based on results.
- Ensure Data Quality: High-quality data is crucial for accurate predictions.
- Train Personnel: Employees must understand and effectively use PdM tools.
- Regularly Update Models: AI and machine learning models should be refined continuously to improve accuracy.
Conclusion
Predictive maintenance is a game-changer for the chemical, oil, and gas industry, offering significant benefits in terms of efficiency, cost savings, and safety. By harnessing the power of IoT, AI, and big data analytics, companies can transition from reactive to proactive maintenance strategies, ensuring long-term sustainability and competitive advantage. As technology continues to evolve, predictive maintenance will become an essential component of modern industrial operations.